Table Of Content
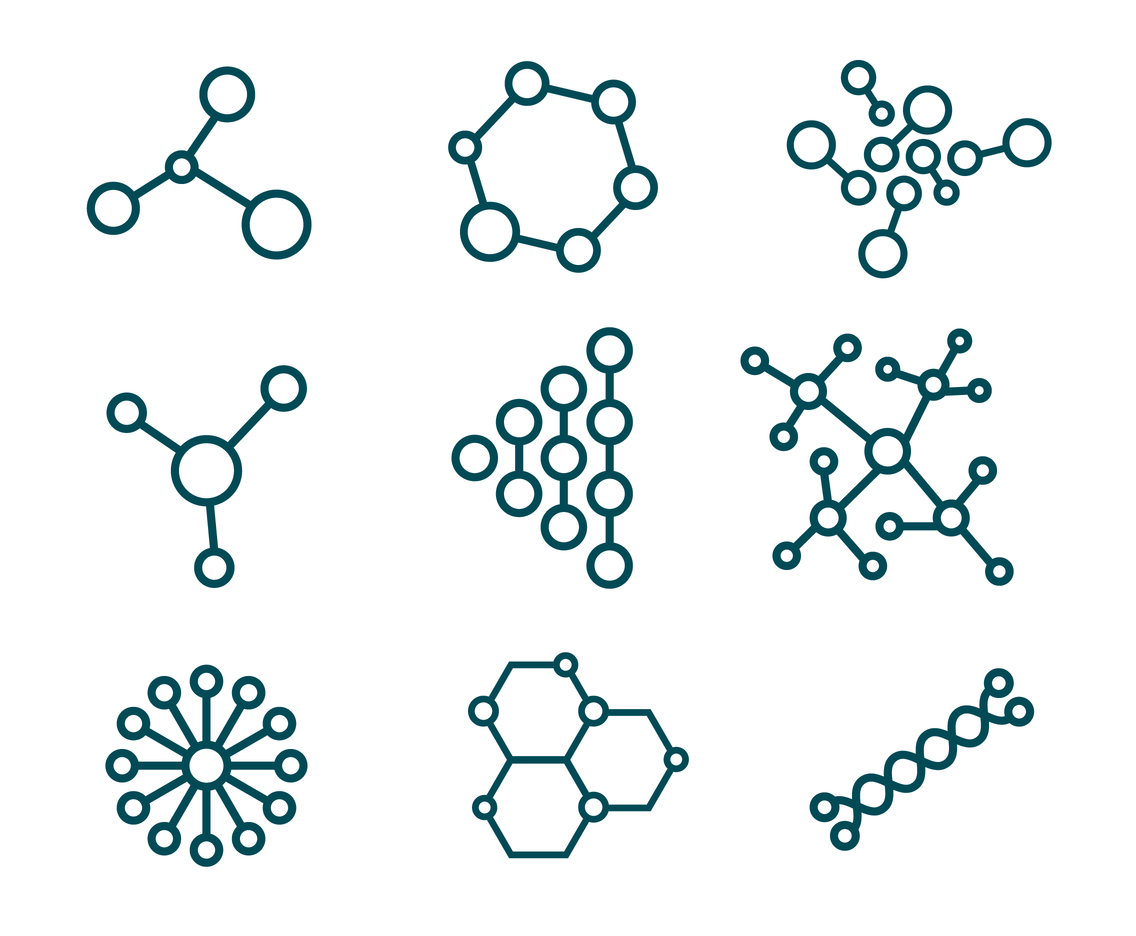
Efficiently using such representations with robust and reproducible ML architectures will provide a predictive modeling engine that would be ethically sourced with molecules metadata. Once a desired accuracy for diverse molecular systems for a given property prediction is achieved, it can routinely be used as an alternative to expensive QM-based simulations or experiments. In the chemical and biological sciences, a major bottleneck for deploying ML models is the lack of sufficiently curated data under similar conditions that is required for training the models. Finding architecture that works consistently well enough for a relatively small amount of data is equally important. Strategies such as active learning (AL) and transfer learning (TL) are ideal for such scenarios to tackle problems [129,130,131,132,133].
SMILES-based models
Two examples of the process of keeping the shape of the initial seed molecules while exploring the training data of the properties of S1. (b) Schematics of the change in the molecular orbital energy when S1 is increased and decreased. R.G.P. and A.M.B. conceived the original idea and designed and supervised the research project.
MilliporeSigma Launches AI Solution for Integrating Drug Discovery and Molecule Synthesis - Pharmaceutical Technology Magazine
MilliporeSigma Launches AI Solution for Integrating Drug Discovery and Molecule Synthesis.
Posted: Fri, 08 Dec 2023 08:00:00 GMT [source]
Crafting molecular architectures with guided diffusion
However, adding the extra structural constraints in GVAE may cause the unnecessary waste of computing and time. Inspired of the attribute grammar, Dai et al. [50] proposed to introduce the stochastic lazy links into attribute grammars which achieved on-the-fly generated guidance for both syntax and semantics check. Deep generative models have been an upsurge in the deep learning community since they were proposed. These models are designed for generating new synthetic data including images, videos and texts by fitting the data approximate distributions. In the last few years, deep generative models have shown superior performance in drug discovery especially de novo molecular design.
Source Data Fig. 3
Mechanism of small molecule inhibition of Plasmodium falciparum myosin A informs antimalarial drug design - Nature.com
Mechanism of small molecule inhibition of Plasmodium falciparum myosin A informs antimalarial drug design.
Posted: Mon, 12 Jun 2023 07:00:00 GMT [source]
CD-learning is an approximate learning approach and has been extensively used for training energy-based models60, so it is chosen for comparison against the energy-based model trained with the quantum generative approach. Specifically regarding organic molecules, two major challenges of EDM are to (1) preserve the chemical validity of evolved molecules and (2) choose the best-fit individuals in each generation efficiently and accurately according to the fitness function. To address the first challenge, heuristic chemical knowledge is generally incorporated. Molecules expressed as graphs or ASCII strings evolve according to user-defined rules, such as adding, deleting, and replacing atoms, bonds, and substructures under chemical constraints. Notably, not only the fragment structures that serve as building blocks but also their attachment points are specified in advance based on previous experience.
True shape of lithium revealed for the first time in UCLA research
The grammatically incorrect SMILES strings are deleted in the inspection step. Synthesizing and characterizing small molecules in a laboratory with desired properties is a time-consuming task [1]. Until recently, experimental laboratories have been mostly human operated; they relied completely on the experts of the field to design experiments, carry out characterization, analyze, validate, and conduct decision making for the final product.
6. Protein Target Specific Molecular Design
In the following, we briefly discuss the main component of the CAMD, while reviewing the recent breakthroughs achieved. Most of models employ the evaluation metrics from various aspects as following. Bickerton et al. [73] utilized the concept of desirability called the QED to measure drug-likeness. And Fréchet ChemNet Distance (FCD) [95] is a measure of distribution between training sets and generated molecules. That |$\log $|P is a particular descriptor estimates the octanol–water partition coefficient.
Separating science fact from fiction in Netflix’s ‘3 Body Problem’
All authors contributed to the study design, analysed the data and jointly wrote the manuscript. VONDOM is a leading company of avant-garde outdoor furniture, planters, lamps, and rugs for modern indoor & outdoor residential and commercial spaces. VONDOM has worked with renowned international designers and architects like Fabio Novembre, Stefano Giovannoni, Eugeni Quitllet, Ora Ïto, Ross Lovegrove, Karim Rashid, Javier Mariscal, and others. Quan Zou is a professor at University of Electronic Science and Technology of China.
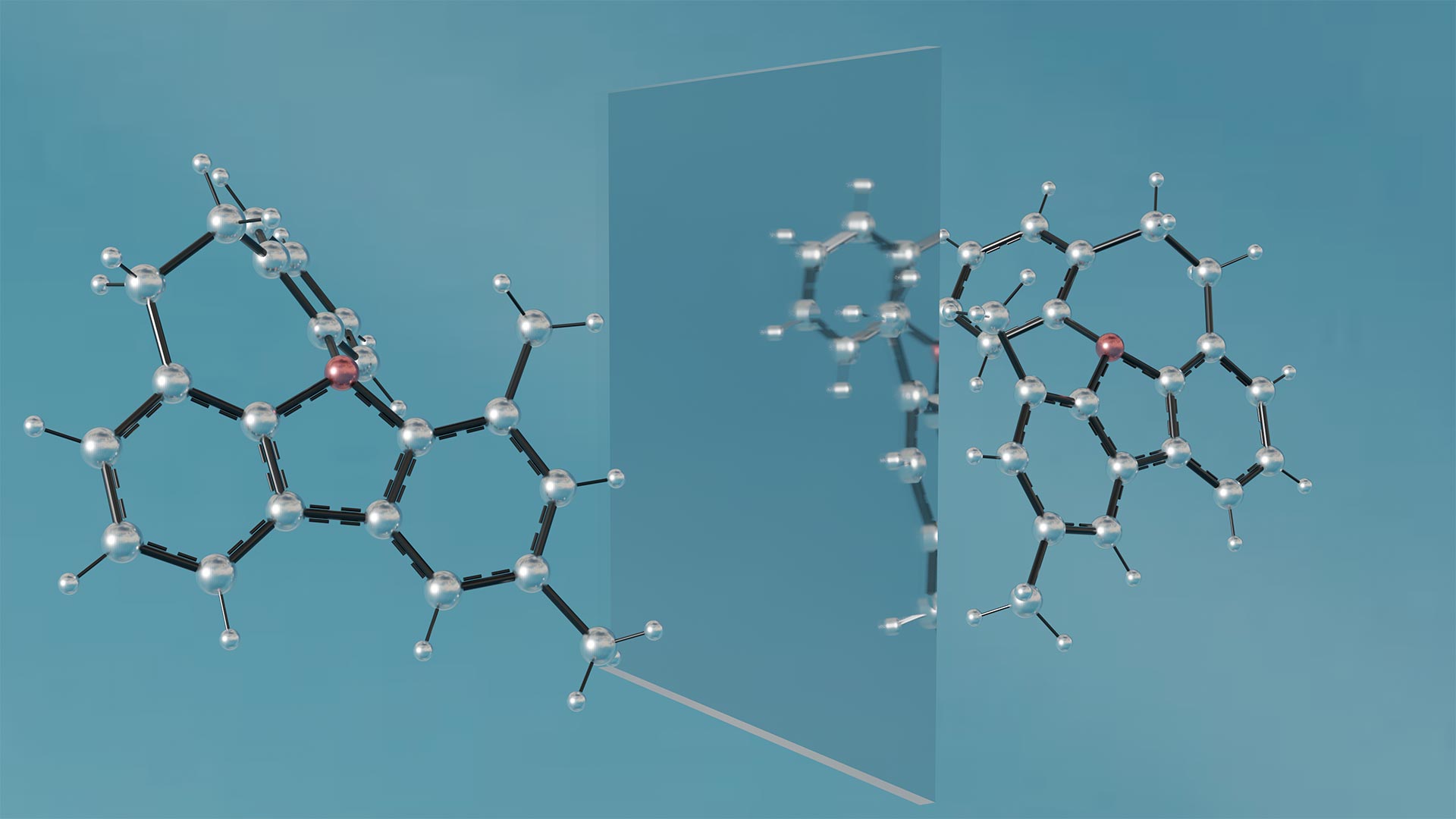
QC-assisted molecule generation framework
The deep learning models learn implicit knowledge from this rich library of materials and successfully guide the automatic evolution of seed molecules without heuristic intervention. Another series of methods, flow-based generative models [45, 87, 88], have been applied for image generation and have recently begun to obtain attention in the molecular generation community. With the help of normalizing flow, the flow-based generation models explicitly learn the data distribution which are consist of invertible transformations. The flow takes an initial variable as input and converts it into a variable with an isotropic Gaussian by repeatedly using the change of variable rule, which is similar to the inference procedure in an encoder of VAE [89]. Non-linear independent components estimation (NICE) [45] was the first normalizing flow architecture which showed satisfying performance on the mixed national institute of standards and technology (MNIST) database and was applied for inpainting. It just roughly stacked fully connected layers so that flow-based models needed to be explored further.
During the follow-up work, RealNVP [87] and Glow [88] yielded unusually brilliant results and became strong performers in the field of generative models. The training of models in machine learning is based on the data, hence we focus on the datasets involved in de novo molecular design here. Specifically, we divide the datasets involved in the typical molecular generative models into the following categories. The researchers trained their model on 250,000 molecular graphs from the ZINC database, a collection of 3-D molecular structures available for public use. They tested the model on tasks to generate valid molecules, find the best lead molecules, and design novel molecules with increase potencies.
Moreover, traditional generative models based on data-driven approaches have limited ability to design new molecules with properties that are not included in the training datasets. In contrast, the proposed method can be designed to produce a new group of candidates by repeating the generation and calculation in that direction even if the molecules with the desired range of chemical characteristics are not included in the training data. Most of current models for molecular generation draw lessons from existing methods in computer vision and natural language processing that do not develop novel models from the perspective of this field. While molecules imitate the representation of images and texts, the generation of images and texts is fault-tolerant. From this aspect, designing unique models and appropriate representations belongs to molecules are warranted.
A lack of accurate, ethically sourced well-curated data is the major bottleneck limiting their use in many domains of physical and biological science. For some sub-domains, a limited amount of data exists that comes mainly from physics-based simulations in databases [25,26] or from experimental databases, such as NIST [27]. For other fields, such as for bio-chemical reactions [28], we have databases with the free energy of reactions, but they are obtained with empirical methods, which are not considered ideal as ground truth for machine learning models.
Domain-aware artificial intelligence has been increasingly adopted in recent years to expedite molecular design in various applications, including drug design and discovery. Recent advances in areas such as physics-informed machine learning and reasoning, software engineering, high-end hardware development, and computing infrastructures are providing opportunities to build scalable and explainable AI molecular discovery systems. This could improve a design hypothesis through feedback analysis, data integration that can provide a basis for the introduction of end-to-end automation for compound discovery and optimization, and enable more intelligent searches of chemical space.
No comments:
Post a Comment